ERC特刊征稿|AI for Model and Data Integration in Hydrology
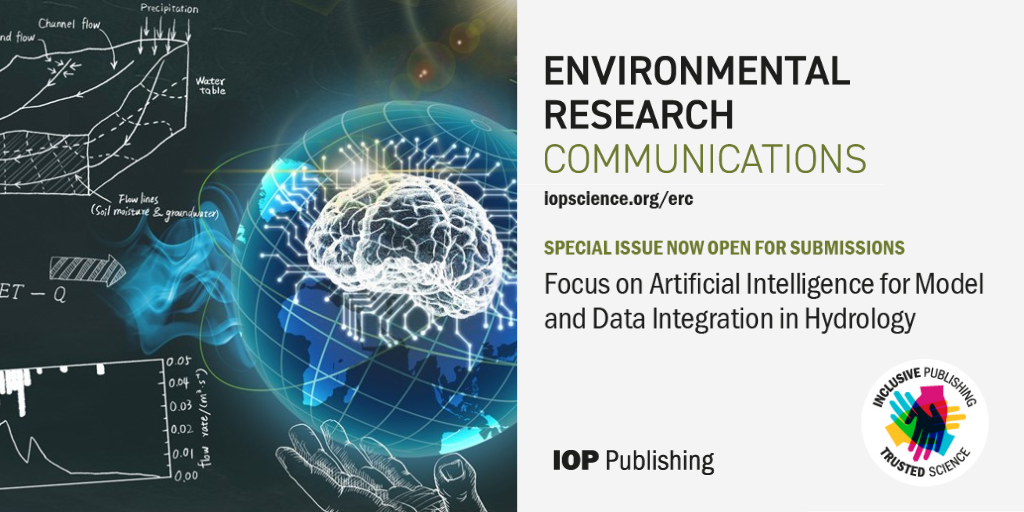
特刊详情
客座编辑
- 史海匀,南方科技大学
- 郑一,南方科技大学
- Chaopeng Shen,美国宾夕法尼亚大学
- 王大刚,中山大学
- 姜丽光,南方科技大学
- 刘苏宁,南方科技大学
主题范围
- Recent AI advances contributed to the field of hydrology
- Promising use cases of AI in hydrological modelling and data integration
- The next generation of applications in hydrology
The Guest Editors particularly welcome contributions that may address the supplementary and complementary role of process-based models and machine learning with respect to the others and also their integration.
The Guest Editors also welcome open call proposals from the community. If you believe you have a suitable research article or topical review in preparation you can send your pre-submission query either to the journal publishing team (erc@ioppublishing.org) or to one of the Guest Editors listed above.
投稿流程
特刊文章与ERC期刊常规文章遵循相同的审稿流程和内容标准,并采用同样的投稿模式。
有关准备文章及投稿的详细信息,可以参阅:https://publishingsupport.iopscience.iop.org/journals/environmental-research-communications/。
作者可登入期刊主页进行在线投稿,选择“文章类型”(Letter/Paper/Topical Review),并在“选择特刊”的下拉框中选择“Focus on Artificial Intelligence for Model and Data Integration in Hydrology”。
投稿截止日期:2024年3月31日。
期刊介绍
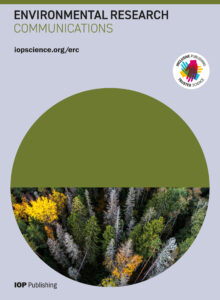
- 2022年影响因子:2.9 Citescore: 3.6
- Environmental Research Communications(ERC)是一本开放获取期刊,涵盖与环境研究相关的所有领域,包括跨学科和多学科的研究。ERC发表推动该领域知识的所有研究结果,包括增量研究、负面结果、无效结果、案例分析、区域性研究和复制研究。