世界量子日系列访谈|MLST期刊编委王磊研究员
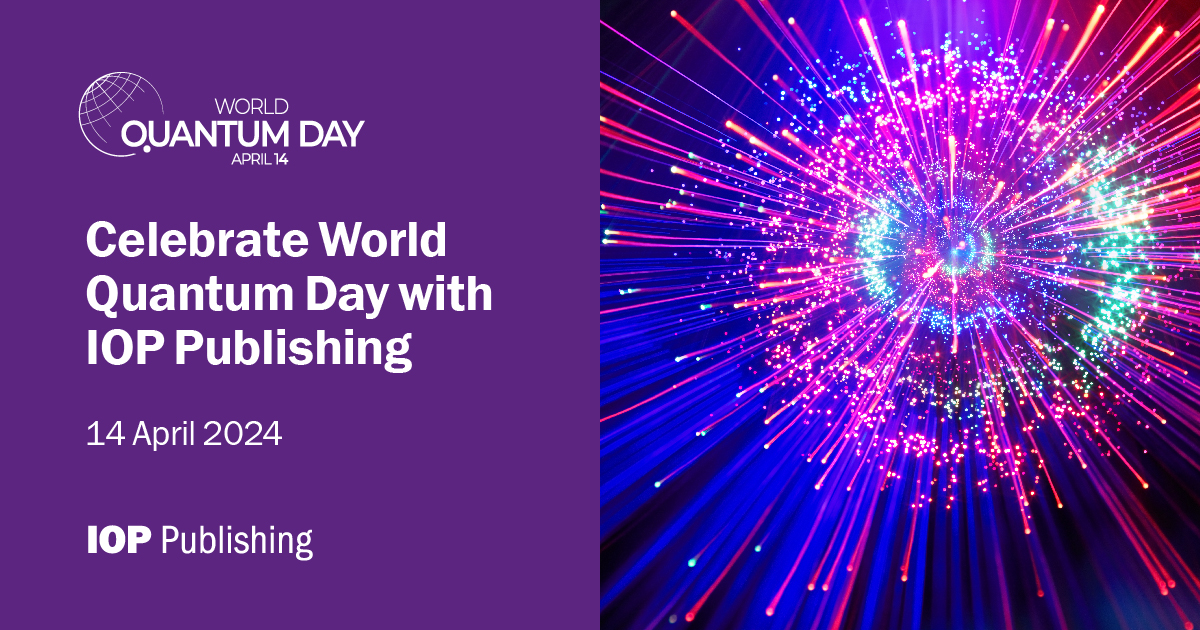
在今年世界量子日来临之际,IOP出版社邀请了多位量子科学领域的编委进行一系列访谈。本次,我们采访了Machine Learning: Science and Technology(MLST)期刊的编委、中国科学院物理研究所的王磊研究员,让我们一起看看他对量子研究领域发展的见解吧。
访谈详情
1. 您目前的研究重点是什么?
My research focuses on in interface of generative AI and condensed matter physics.
Generative AI aims at modeling, learning, and sampling from high-dimensional probability distributions. It has a natural connection to many topics in condensed matter physics which focuses on emergent phenomena of many-particle systems in Nature.
In the past years, I have worked on minimizing Nature’s cost function — the variational free energy — with the help of generative models.
At the moment, I am crafting a generative model for crystalline materials with space group symmetries.
2. 您认为到目前为止,量子科学领域最大的进步是什么?
To me, the biggest advancement in quantum science is the combination of a few very simple quantum mechanical principles with computational tools to predict the complex world, ranging from semiconductors, superconductivities, magnetism, chemistry, etc.
3. 在您看来,量子科学与技术领域的下一个重大突破可能是什么?
Hard to predict. In my biased view, I would bet on an agent that automates the exploration of the materials space, which may give better superconductors.
4. 您认为MLST期刊在支持该领域研究方面起到了什么作用?
It creates a town square for interdisciplinary works to be displayed to scientists with vastly different backgrounds. Fast dissemination of information, especially across language barriers between fields is important to today’s technical advances. MLST has done a great job.
编委介绍
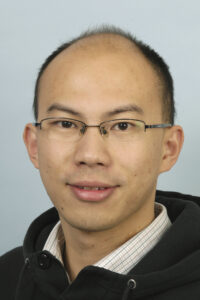
王磊 研究员
中国科学院物理研究所
- Lei Wang got his Bachelor’s degree from Nanjing University in 2006 and Ph.D. from the Institute of Physics, Chinese Academy of Sciences in 2011. He did postdoctoral research on computational quantum physics at ETH Zurich in the next few years. Lei Wang joined the Institute of Physics in 2016. His research interest is at the cross-section of machine learning and quantum many-body computation.
量子领域最新资讯
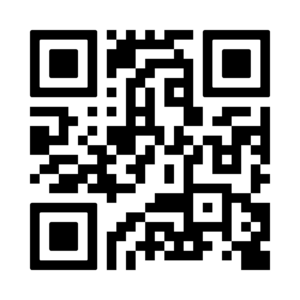
期刊介绍
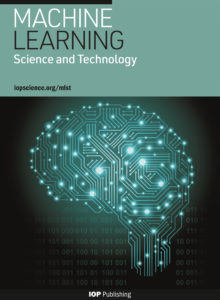
- 2022年影响因子:6.8 Citescore:7.1
- Machine Learning: Science and Technology (MLST)是一本跨学科期刊,致力于发表智能机器在物理、材料科学、化学、生物学、医学、地球科学、天文学和工程学等多学科领域的应用和发展。涉及领域包括:物理学和空间科学;设计和发现新材料和分子;材料表征技术;模拟材料、化学过程和生物系统;原子和粗粒度模拟;量子计算;生物学、医学和生物医学成像;地球科学(包括自然灾害预测)和气候学;模拟方法和高性能计算。同时,也包括机器学习方法在概念上的新进展:新的学习算法;深度学习架构;核心方法;概率和贝叶斯方法;生成方法;强化和主动学习;经常性和基于时间结构的方法;神经启发方法(包括神经形态计算)。